Revolutionizing procurement: Leveraging data and AI for strategic advantage
In today’s tumultuous business landscape, where price volatility, geopolitical tensions, and sustainability imperatives converge, one function stands at the forefront of navigating these complexities: procurement. In this context, the procurement function will act as a strategic lever for value protection and creation.
Procurement sits at the confluence of huge quantities of data , flowing from within the organization (for example, spend, demand patterns, specifications) and from without (suppliers, market insights databases, and the wider web). Today’s digitized, connected organizations must tap into this data and develop new tools to make faster, better sourcing decisions. Mastering the data will empower procurement teams to achieve strategic objectives that go far beyond traditional cost, quality, and delivery metrics.

What data can deliver
Better data can support activities and decisions across the sourcing life cycle, from the development of category strategies and the assessment of potential suppliers to the execution of negotiations and ongoing supplier performance management. Done well, that can increase the pipeline of value creation initiatives by up to 200 percent. Let’s look at five areas where data will have the most impact:
- Optimizing spend and demand. With the application of AI and generative AI (gen AI) technologies, category management can be automated and accelerated in multiple ways. First, spend categorization algorithms can create cleaned spend cubes seamlessly. Second, demand forecasts and optimization will see a step change in accuracy, making sourcing, demand, and supply chain control and optimization much more relevant. Similarly, gen AI interfaces allow procurement leaders to interrogate spend, market, or specifications data, asking questions regarding, for example, the share of spend exposed to a particular climate or geopolitical event, the should-cost increase due to oil price fluctuations, or the possible alternative sources to a supplier in distress. For standardized items with highly competitive markets such as transportations or temporary labor, buyers would not need to interfere, leaving bots to make trade decisions autonomously based on predefined objective functions. Gen AI can also be used to automate contract generation for the category, as some airline companies are starting to do at scale. Another disruption will touch supply market analysis and strategy optimization, identifying sources that expose the company to high risks and automatically finding alternative sources within a defined price range and lead-time. Finally, companies can use machine learning to analyze usage patterns and forecast demand to automatically generate sourcing scenarios and strategies based on inventory-level intelligence, especially for frequently bought items.
- Managing external drivers of profitability. Procurement teams will be able to combine internal data with external market reports and databases and use machine learning algorithms to uncover patterns and trends in commodity prices. Chief procurement officers and category managers will rely on such predictions to stay at the forefront of industry profitability with real-time transparency on price volatility exposure. They will be able to dynamically compute the should-cost of their most volatile commodities and negotiate with suppliers based on facts. Procurement will also be able to assess the impact of any variation in input prices on the product margins, and analyze multiple scenarios to define the right actions to protect those margins. Reactive actions may include shifting to alternative approved recipes or value chains, adjusting planning or stock levels, financially hedging commodities, or passing pricing changes to the customer. For example, a consumer company developed a price forecasting model that was combined with a hedging optimizer to recommend monthly the volume of palm oil derivatives to hedge for. Mid- and long-term actions may include optimizing contracting strategy by adjusting contract lengths and start dates in negotiations with suppliers (for example, spot buy versus fixed price for a few months), redefining supplier regional footprint by taking advantage of price evolution differences across countries, or obtaining cost advantages through strategic agreements with selected Tier-N suppliers, including partial acquisition or vertical integration with value chain players obtaining disproportional margins.
- Managing supplier performance. Digital dashboards can combine contract, invoice, and supplier delivery performance data to provide a comprehensive picture of supplier adherence to service-level agreements. By providing early warnings of performance deviations, these systems can steer operational interventions or supplier collaboration projects. Parametric cleansheet tools can automatically calculate the should-cost for thousands of items, helping companies optimize specifications, and allowing them to conduct robust, fact-based negotiations with their suppliers. Gen AI technologies are changing supplier management too, with the emergence of automated tools that can produce smart intelligence on supplier risk profiles based on public data such as social media reports. Gen AI can also help procurement personnel optimize their dialogue with suppliers, for example, by automating the creation of negotiation scripts, reports, emails, and contracts.
- Managing supply risks. In best practice by 2030, procurement will be equipped with a digital twin of their supply chain, modeling all nodes across the globe, from sub-tier suppliers of raw materials and their direct suppliers to the internal manufacturing network, the customers, and all the logistic channels connecting these nodes. These digital twins will be developed by combining two approaches. Material flows from tier-one suppliers will be mapped in collaboration with suppliers, while web data mining will be used to close data gaps by building a picture of flows from across tier two to tier-n. Each node will have a near-live view of the associated supply risk, cost, and carbon intensity, computed based on logic that allows filtering of signals by their actual risk and the availability of mitigation actions. Procurement will not only have a thorough understanding of the present state of its supply chain but will also be able to simulate the risk level given forecasted business growth, the occurrence of risk events, and the effect of mitigation actions. The digital supply chain twin will allow procurement to assess the implications of any type of change or disruption in highly complex and interconnected value chains and react to adverse events much earlier. In addition, teams that adopt this technology will react faster than their peers to changes in supply signals and, as a result, have the right product at the right place and at the lowest cost and carbon footprint.
- Leading on sustainability. Sustainability-centric data and effective analytical tools are vital as companies strive to meet demanding goals for carbon reduction, pollution prevention, and the elimination of unfair labor practices in the supply chain. In terms of environmental sustainability, procurement data based on expenditure can be utilized to estimate the baseline for carbon emissions within the supply chain. This approach has been adopted by many companies to evaluate their upstream Scope 3 emissions. Enhancing bilateral carbon transparency between suppliers and customers necessitates additional efforts, however, including collaboration with suppliers to establish product-level emission reporting (for instance, consumption-based emission factors) and sharing standards through existing procurement data infrastructure. Integrating these sustainability metrics with procurement data and advanced analytics can assist companies in engaging with, evaluating, and monitoring the sustainability progress of their suppliers. Consequently, the approach can help companies reduce their supply chain emissions by selecting products or suppliers that align with their sustainability goals.
The data-driven procurement revolution is already under way. The World Economic Forum (WEF) Global Lighthouse Network is a diverse community of manufacturers united by their leadership in the use of Fourth Industrial Revolution technologies to transform factories, value chains, and business models. Two recent members of the network earned their place by transforming their procurement operations with digital and AI technologies.
Pharmaceutical company Sanofi applied should-cost modeling to inform make-versus-buy design across multiple categories, achieving an average 10 percent reduction in spend. An advanced analytics platform reduced the time required to evaluate tenders by two-thirds, and digitally enabled negotiations helped it increase the savings achieved by 281 percent.
Teva Pharmaceuticals used analytics-driven procurement supported by spend intelligence and an automated spend cube to achieve a more-than-tenfold improvement in supply resilience. The company’s smart spend category creation systems cut the time required to develop category strategies by 90 percent. Teva’s global procurement unit, based in Amsterdam, is the main contributor to its ambitious gross margin improvement program.
Significant challenges remain
Senior procurement leaders understand that more-effective use of data is vital. Yet many procurement functions still struggle to transform themselves into data- and technology-driven organizations. In a recent McKinsey survey, CPOs highlighted three key problems that they believe are holding back their digital ambitions: issues with data quality and access, lack of clarity over the business case for new digital or AI applications, and difficulty driving adoption of the new tools at scale.
Data quality and access challenges. CPOs expect data, analytics, and gen AI to play a core role in every business decision by 2030, but respondents to our survey admit that their data infrastructure is not ready to support this ambition. Twenty-one percent say their data infrastructure maturity is low, with less than 70 percent of spend data stored in one place. An additional 30 percent think they have average levels of data maturity, and even those who have implemented systems to give them a single source of truth for all spend data admit that this data is not cleaned and categorized. These systems may also lack important information from outside the procurement function, such as quality or specification data, or external data from suppliers, customers, and the wider market.
Difficulty articulating the business case. Procurement teams also find it difficult to secure funding for analytics and AI projects, often due to the lack of a compelling business case. This challenge is typical in organizations that follow a “technology-back” approach, that is, selecting software and solutions without a clear link to business value creation opportunities.
Low levels of adoption. Organizations that overcome the first two challenges often run into the third. Even when they have built a business case and proved the effectiveness of a digital use case in tests, they find it difficult to embed its use in their core processes and teams’ ways of working across the organization. This is a common challenge in data analytics transformations regardless of business function, leaving many organizations stuck in pilot purgatory. It is especially common in procurement, where teams are often focused on delivering quarterly results, or submerged by short-term obligations, and do not take the time to understand and adopt new technical solutions.
The recipe for success at scale
Scaling a data analytics transformation is what differentiates Lighthouses from the rest of the pack. Companies that have achieved this tend to get a few vital things right. They focus on a small number of high-value digital and AI use cases. They build and, crucially, own a robust data infrastructure to support those use cases. And they spend as much time thinking about people and processes as on technology: adapting their core business processes and operating model, upskilling and reskilling their people, and steering change across the organization.
A focus on high-value use cases
Many procurement organizations have road maps that aim to deploy fifteen or more data products every year, including the rollout of complex data architecture and end-to-end suites. In our experience, however, organizations have limitations on their capacity to test, validate, industrialize, and scale such a volume of technical solutions. Similar limitations apply to the IT function’s ability and capacity to steer and industrialize new tools, and category and buying teams’ ability to integrate those tools while delivering on their annual business objectives.
Companies that have been able to scale analytics successfully have focused their annual road maps on a prioritized set of five or six technical solutions selected based on the value potential each can create and how each addresses core business questions and users’ needs. The Pareto principle applies, with a handful of data products delivering 60 percent to 80 percent of the value at stake and making investments net positive in the eight-to-twelve-month range.
One WEF Lighthouse organization prioritized six use cases for its procurement analytics transformation: category analytics, parametric cleansheets, predictive pricing, and digital trackers to monitor input costs, supplier performance, and supply risks. Using just those use cases, the company was able to double the value creation opportunities identified by the procurement function.
Dedicated data platform/domain and dedicated technical resources
An encouraging number of companies have started to disrupt the way data transforms procurement by enriching their spend data with a mix of AI-powered data categorization and rigorous master data management practices. This allows them to build data models that integrate a comprehensive set of relevant data sources, both internal and external, such as market insight databases.
Leading organizations create their own procurement data model with a dedicated team. Rather than attempting to fix all data at once, leaders focus on the data they need for high-priority use cases and work backward from there. This helps every data component that is processed create value for the organization, versus ingesting diverse data sets before evaluating their uses. And while systems exist to cleanse data, strengthening data governance processes remains a priority.
Equally important is partnership with the IT function from the beginning. Driving a holistic analytics transformation is as much a technology transformation as a business practices transformation. It requires the support of the company’s best technical architects and engineers to succeed. Partnering with IT and digital at the outset is critical to ensure all design choices are made in line with best practice, and to get sufficient technical capacity and expertise to build the required data models and pipelines.
Putting users at the center
Considering user-centricity from day one is crucial to driving fast adoption throughout the life cycle of a data product. This starts by ensuring the needs, pain points, and preferences of procurement professionals are understood early on. Unnecessary features that drive complexity and ultimately deteriorate user experience must be avoided. In addition, the user interface needs to be intuitive and easy to navigate, ensuring that users can quickly grasp the functionality and benefits. It is important to consider existing procurement systems and processes so that new data products are integrated seamlessly, reducing disruption for users and the need to mitigate processes with change management.
Meanwhile, communication and training are key to the successful launch of data products. Clearly articulating how new data products can enhance efficiency, streamline processes, and improve decision making motivates early adoption. This communication needs to be bidirectional, actively listening to users, incorporating their input, and upgrading features, while conveying a clear change narrative from CPOs and their leadership teams.
Talent and skills
Procurement teams don’t typically have enough people skilled in data, analytics, and AI to support their digital ambitions. Our survey highlighted a direct correlation between the level of advancement of an organization and its share of available analytical resources. Best-in-class companies place 22 percent of procurement employees in analytics teams. This suggests that companies will need to invest and increase the number of data profiles available to scale through external hiring of data-savvy profiles or reskilling of existing teams.
Track impact and manage performance
Leading organizations invest resources to keep their data transformations on track. Typically, they set up a transformation office that monitors progress versus the initial road map and tracks impact delivery rigorously, raising flags when solutions underdeliver. This allows procurement to step back and analyze what may not be going as planned, enabling course correction. One WEF Lighthouse is tracking value creation and the number of users of each data product on a weekly basis, reviewing with the CPO monthly to ensure the right progress and to unlock the pockets of resistance early on. In the same organization, the impact delivered on categories by each data product is tracked and recorded in the value tracking system, to ensure full transparency on which data products work and can be scaled, versus which ones are stalling and require support.
We have seen a leading company interrupt the development of a data product that required heavy manual work to compile product specifications data, for which the organization and technical architecture was not ready. In another example, a leading consumer packaged goods player entrenched in developing digital design-to-value capabilities identified early on that business partners had low ownership of the value creation ideas it generated. Stepping back, it adapted its approach by bringing the business to the core of solution deployment.
From vision to transformation
Turning the procurement function into a data-driven, AI-enabled organization is a process of transformation that typically takes six to eighteen months to make a step change. Every successful transformation requires vision, ambition, and sustained commitment from senior leadership. It also depends upon teamwork, engagement, and excitement from across the organization. Any CPO embarking on such a journey should begin as they mean to continue: by collaborating with internal and external stakeholders.
As a first step, engage with stakeholders across the business to understand what they need from a high-performing procurement function, and where their major pain points are today. That will help procurement get its priorities right, allowing it to identify the data products that will deliver significant value quickly. Technology partners will be a second key group of collaborators. They include the organization’s internal IT function and external suppliers of data platforms, AI technologies, and analytical tools.
Armed with a clear picture of business needs and potential solutions, procurement can revise its technology road map. It should do that with twin objectives in mind: early implementation of AI and analytics solutions that create value, while building the foundations of a data platform that will meet the organization’s long-term needs. Quick wins from the first use cases are critical in building momentum for the transformation. By showing end users and business leaders what data and analytics can deliver, they help foster excitement and drive engagement across the organization.
And, as they begin to deploy those high-priority solutions, procurement leaders should keep another group of collaborators at front of mind: the procurement teams who translate data-driven insights into value for the business. Focusing on the adoption of AI technologies from day one helps procurement build solutions that work better, scale faster, and create more value for the organization.
Aasheesh Mittal is a partner in McKinsey’s Washington, DC, office; Charles Cocoual is a partner in the Brussels office; Mauro Erriquez is a senior partner in the Frankfurt office; and Theano Liakopoulou is a partner in the Paris office.
Explore a career with us
Related articles.
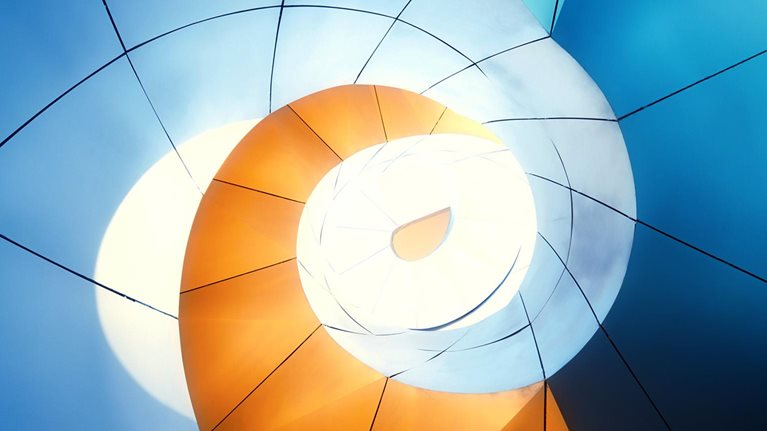
A new era for procurement: Value creation across the supply chain
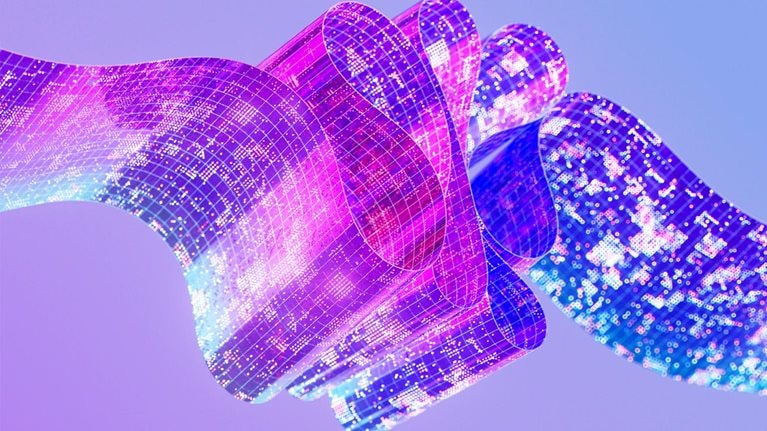
Generative AI in operations: Capturing the value
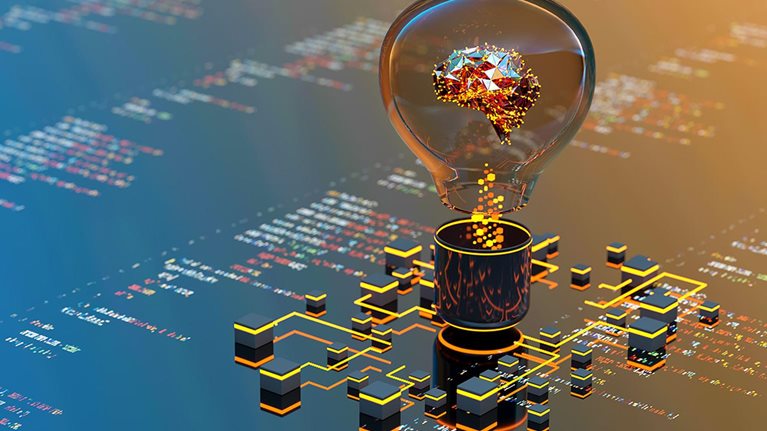
Making the leap with generative AI in procurement
Intelligent procurement
- Call For Change
- When Tech Meets Human Ingenuity
- A Valuable Difference
- Meet The Team
- Related Capabilities

Call for change
As procurement functions realize the value their data and their relationships with third parties can add to an organization, they are trying to shift from their traditional offering of transactional compliance to being a business partner that can help their companies grow their bottom line.
Making this transition means standardizing, simplifying and automating the elements of traditional procurement to create a frictionless buying experience. This accomplishment will help move procurement functions toward enabling intelligent procurement.
As Accenture makes this shift itself, we recognized the need for a strong data and analytics foundation. Our Procurement Plus organization—so named with the word “Plus” added to reflect the shift in delivering value above and beyond what is expected of traditional procurement—is developing a data strategy and program to empower us to be more data driven in analysis, information tracking and decision making. We recognize this is a journey, and like all transformations today, the journey is best undertaken in an agile way.
When done right, a data and analytics strategy is a powerful tool that enables Procurement to be a valuable business partner delivering real change as our Procurement Plus did with Accenture’s closed-loop spend management (CLSM) approach . CLSM is a new, data-driven operating model for managing the source-to-pay life cycle that targets indirect and direct costs for meaningful and sustained transformation.
Procurement Plus by the numbers
procurement spend
invoices paid
ventures & acquisitions work projects
US spend with diverse suppliers
As of Accenture fiscal 2020 year end. *On top of standard procurement FTE it includes more than 500 FTE in Accounts Payable and our internal contractor managed service provider.
When tech meets human ingenuity
In our attempt to deliver a data strategy, we started small with the focus on delivering value. This approach allowed us to gain support for a larger investment to turn us into the intelligent procurement function that we aspire to be.
Digitizing processes to create access to data
Our agile journey to transform procurement began with Accenture’s move to SAP Ariba Buying and Invoicing and the deployment of the Guided Buying capability across Accenture to improve the buying experience. Our Procurement Plus and global IT organizations have continued to collaborate to enhance our capability. We are transforming content, digitizing procurement processes, building automations and intelligence on SAP Ariba, all on the journey to developing intelligent procurement.
Throughout this time, we’ve gained experience in addressing data and developing analytics solutions along with Accenture’s Applied Intelligence organization. Together, we have developed several advanced analytics solutions that help us address specific issues today.
Setting the foundation for advanced analytics
The advanced analytics solutions we have developed include:
Ariba procure-to-pay process improvement. We are using Celonis , a process mining and execution management solution, to identify and remove bottlenecks in the procure-to-pay process and improve the quality of execution. In so doing, we are optimizing standardization at scale. The data visualization capabilities of Celonis are helping us to develop deeper insights, allowing us to have fact-based conversations and highlighting similarities or differences of workflows, categories, or countries that are impacting global process turnaround time. We are leveraging the newer workbench and execution management capabilities to automate the execution of activities to confirm we can offer a higher level of operational service without increasing head count. This is a powerful enabler for change.
General ledger recommendations. We are using predictive analytics to identify and assign the financial data on requisitions and non-purchase order invoices. This capability better prepares buyers who are less familiar with accounting to be more accurate with their purchases, improving their user experience and significantly streamlining downstream accounts payable accuracy, time and cost.
Predictive contractor fulfillment. This tool uses predictive analytics that draw on historical information to help Accenture determine whether a contractor request can be filled. It also helps to assess the quality of the requirements and to view trends on cancellations by categories, clients and geographies. The tool allows our contractor onboarding teams to apply their time where it’s most valuable, delivering huge time savings in effort and higher satisfaction from suppliers in the quality of requests that they receive.
360-degree supplier relationships view. By integrating data about Accenture’s family of suppliers with Dun & Bradstreet services, this tool helps us understand the full scale of the relationship and partnership Accenture has with our suppliers, which is the key to gaining better insights as we partner with them.
Metrics at users’ fingertips. We have created and integrated targeted dashboards across the procure-to-pay and accounts payables areas to cascade to any user. The dashboards present performance against service level agreements (SLAs) and other business outcomes and key performance indicators (KPIs). They also derive data-driven, actionable insights and determine high-impact opportunities for process and key outcome improvements.
Creating a holistic, robust digital strategy
Developing these specific solutions has allowed our Procurement Plus team to reach a mature stage in advancing our data strategy and deliver valuable use cases that showcase the return on investment to leadership. This demonstration has enabled us to begin the next phase of execution on our data and analytics journey.
Data Strategy. Our approach now consists of two layers: the first is the normalized movement of data to enable a simplified user experience and the second is the positioning of data in a shared location so that it can be consolidated and consumed. With this central storage in place, procurement data can be analyzed effectively cross-process. This ability allows us to gain new insights and be able to answer future, unanticipated questions based upon new business disruptions.
This new data-centric environment also allows us to accelerate development of more advanced solutions supporting the connected buying experience for our people, comprehensive frictionless third-party risk management, and intelligent procurement.
Connected experience . To reduce friction for our people, we are developing a “connected experience” for buying that is underpinned by a fully digitized process enabled by data and analytics. We recognized the need to give Accenture people a more effective response to “how do I buy something?” and be navigated through the procurement process.
Our connected experience capability consists of several key components. We are developing a “front door” via ServiceNow to provide users with a single and connected entry for all their procurement needs. Sourcing will be automated and handled through a dashboard giving users updates and reminders from all procurement systems all in one place.
The workflow data architecture will then connect all sourcing activities with the correct buying channel. This architecture will essentially bring our sourcing activities from the front door together with the buying into one central data lake that is curated and integrated. This visibility will confirm, for example, what we bought from a supplier and whether we paid them according to the terms agreed upon during our sourcing and contracting process. These and many other insights will enable us to measure process effectiveness through the total life cycle of procurement.
Third-party risk management. The data journey and the connected experience will support third-party risk management. By capturing the data in a curated fashion, from the time we make a request to buy through our sourcing and contracting activities and the buying process, we can measure the relative risk of any given supplier compared to others, both from a compliance and performance perspective, according to the criteria we set forth on what defines risk. Bringing together more data elements enables us to establish a risk score and gain a full view of each supplier.
Leveraging data to drive intelligent procurement
With the data we now have, we’re able to answer questions that we could never answer before. For example, we can measure whether our respective spend category plans are being implemented as intended. Detailed insights regarding spend and performance will move Accenture toward engaging with suppliers that are the best fit for our categories.
The insights help us confirm that we are engaging with the right suppliers to contribute to Accenture’s innovation and sustainability agenda. The insights also help us to obtain better arrangements, pricing, and quality and help us be smarter with the suppliers we’re engaging with, ultimately driving margin, where possible.
A few example use cases include compliance to contract and payment terms, demand concentration according to category plans, and balance of trade. Yet another example is getting a better sense of the trajectory around contractor needs in relationship to demand trends and costs. All these user scenarios were developed by a structured input/output review of data traversing the procurement processes. The outcomes help to highlight current disconnects in the process and identify the data that will be needed to join the processes automatically and analyze their effectiveness.
A valuable difference
The future of procurement is in intelligence. By unleashing data and analytics as we progress on our program journey, we anticipate gaining new insights to get better at managing the total procurement life cycle while continually improving the customer experience.
Key outcomes from our journey with process analytics:
Celonis Continuously improves the customer experience and effectiveness of internal teams by reducing friction and improving turnaround time
Predictive contractor fulfillment Fulfills contractor requests much faster and more effectively than our previous process and with less manual effort
General Ledger Recommendation Delivers an improved procurement experience and greatly streamlines accounts payable accuracy, time and cost
Connected experience Planned to be more comprehensive and standardized than today in guiding Accenture people through the buying process
“Bringing the right data and steps together in the procurement process will give us enormously valuable insights into how we’re contracting and buying and with which suppliers.” — PATRICIA MILLER , Director of Profitable & Responsible Buying
Annualized working capital benefits delivered by having greater visibility into our pending invoices, as of fiscal year 2021.
Reduction in invoice approval time.
Improvement in request-to-order time.
Meet the team
Tricia Miller
Jim Gradeless
Scott Perkins
Related capabilities, corporate services & sustainability.
These teams are enabling innovation, growth and business continuity for Accenture.
How Accenture does IT
Sourcing and procurement.
Supply Chain
Procurement 4.0 - a digital supply chain case study.
We often hear about Smart Factories, Smart Manufacturing, Plant Automation, etc. Most of the Industrial Digitalization/Digital Transformation and Industry 4.0 applications focus on digitalizing the manufacturing processes, improving production, and shop-floor visibility. However, very few companies talk about making their supply-chain smart or adopting digitalization towards individual aspects of the supply chain.
Digitalization can help improve supply chain performance drastically at a very minimal cost, thereby having a significant impact on the bottom line.
For example, consider an enterprise dealing with import, processing, and distribution of vegetable oil, beverages or any other commodity items, with a revenue of USD 500 Million. Typically, such an enterprise loses USD 7.5 Million to USD 15 Million (1.5% - 3.0% of revenue) through various leaks, pilferages and inefficiencies in the supply chain. The losses could be further attributed to delays, stock-out problems, vendor problems, quality, etc. 40% to 70% of these losses can be eliminated through simple digitalization with almost no additional Capex. More importantly, the payback time of such projects is often less than a month or two.
In this article, I would talk about Supply Chain Digitalization with a focus on the procurement side - Procurement 4.0 . I’ll run through a brief case study of a large process manufacturer in Asia to explain the points in a relatable manner. However, the overall idea remains the same for Discreet industries as well.
At a very high level, this is how a typical supply chain process looks like:

A manufacturer procures the required raw material, stores it as raw material inventory, processes it, and finally the finished products are dispatched through a distribution network. The above representation is a highly simplified view. The entire supply chain process has its own complexities with various stages and processes and involvement of multiple stakeholders and functions (Procurement, Inventory management, Production, Distribution, Finance, etc.). One can only imagine the amount of cross-functional coordination that would be involved and the quantum of data generated during the day-to-day operations.
Let us look at the sourcing side in further detail:

A large process manufacturer - chemical/ oil company, etc., imports crude (e.g., raw vegetable oil) and other raw materials from various countries through a complex procurement mechanism involving multiple stakeholders. The crude may be transported in a mother vessel before being transferred to smaller vessels to finally arrive at the nearest jetty or pipeline of the manufacturer. In another case, it may be further transferred by rail cars to reach the nearest processing plant.
There are various problems a manufacturer might face in the procurement process (sourcing from various places, vendor management, timely delivery, missing/incorrect shipments, quality, transit loss, optimizing costs, etc.). Recently, one of our customers (a large process manufacturing company) was unable to reconcile a lot of their inventory - both on the procurement side, as well as the dispatch side. They were also facing huge losses during transit. The customer approached us for a solution and wanted to use technology to minimize these losses.
We observed that most of the data was being captured manually on paper with no systematic records. Surveyor reports were being generated on paper, tank inventory was being monitored using dip-sticks, and manual recordings were being for the processed oil at dispatch stations.
The data which was indeed available in databases (e.g., vessel-related information - coordinates, charter info, material quantity, etc.) was lying in silos. Even though the company did have a large ERP system installed, it was again a siloed system. No cross-functional oversight and insight was available within the opportunity window. The data was only useful for manual post mortem analysis of the overall gross losses suffered. Neither it was possible to pin-point the problem areas, nor was it possible to intervene in time. We have also seen companies that are not even aware of the cost they incur due to such inefficiencies and missed opportunities.
The below diagram indicates how the current operations looked like and the various data that was being generated.

We observed that there was massive scope for digitalization just on the procurement side itself. Fetching and analyzing data from simple instruments could solve not only the inventory and transit loss related problems but provide visibility into a lot of the other use-cases as well.
As part of the solution to the reconciliation problem, we identified the following areas which could be easily digitalized with minimal requirement for new instrumentation (marked in Yellow & Red Stars):
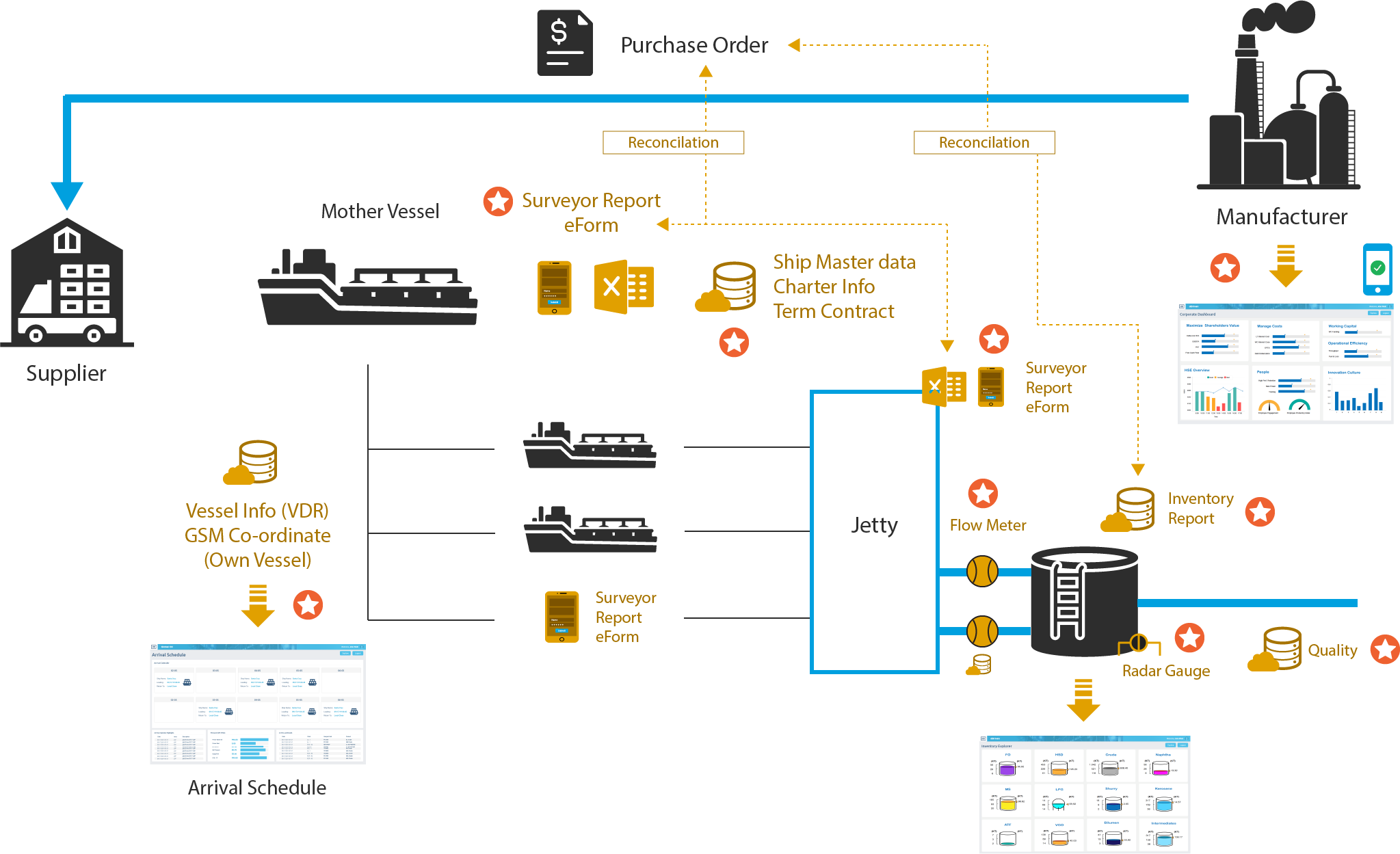
Some of the use-cases which we identified are:
- Once the customer moves away from physical copies of surveyor reports at various junctures to e-forms/online records, the reconciliation of surveyor reports with Purchase Order details becomes extremely easy and can be done in an automated manner with real-time alerts in case of discrepancies
- Complete visibility into transit loss at each stage of the procurement process (from mother vessel till the time it is transmitted to the storage tanks)
- Automated records of actual crude oil received using flow meters
- Data from radar gauges could help in real-time visibility into inventory data as well as oil consumed during any particular period
However, there are a lot more benefits which could be obtained from digitalization of the procurement process:
- Creating optimized sourcing plans as per back-propagated demand – this could further help in optimizing the Working Capital, utilization of processing capacity and availability of final products
- The vessel information data could be used to derive optimal arrival plans and reduce demurrage
- Best suppliers, shippers – By having complete records of who supplied what, the best suppliers (based on on-time delivery, quality of supply, payment terms, etc.) can be easily identified. This could further help in improving the quality and optimizing the manufacturer’s processes.
- Because of real-time availability into inventory data, tank storage could be optimized further. Our customer was using two different types of crude, and one of the pain-points was related to intermixing of these (apparently, if one of the tanks was 5% full, the onsite workforce used to use this tank for storing the other type of oil). With real-time information, this problem could be solved, which would again help in improving the quality of the final product.
- Records of all trucks and data from weighbridges could be further digitalized with automatic reconciliation against purchase orders and alerts in case of discrepancies.
Overall, the potential benefits which can be realized through digitalization are immense and far outweigh the costs of implementing such a project. If used well, the already existing data (lying in multiple siloed systems and manual records) can unleash massive hidden value for the company. In many of the cases, the payback period is as less as one month. Other than this, you get complete visibility into your operations from the touch of a button sitting anywhere in the world.
Given how the world is changing today, I feel digitalization is no longer an option, but an imperative. In the long run, it is only the highly adaptable with a strong willingness to continue improving and adopting the best practices who would continue to survive and thrive.
Connect with dDriven

IMAGES
VIDEO
COMMENTS
One WEF Lighthouse organization prioritized six use cases for its procurement analytics transformation: category analytics, parametric cleansheets, predictive pricing, and …
The journey to procurement digitization. Authored by: Obehi Omobhude, Mariana Loera Solorzano, Cara Sharko, Jason Zannet, Marco Garces, and Biju George. Digital …
Our Procurement Plus and global IT organizations have continued to collaborate to enhance our capability. We are transforming content, digitizing procurement processes, building automations and intelligence on SAP Ariba, all on the …
Longitudinal case studies should be used to better understand the process and transformation of implementing procurement technologies in practice and understand how they are integrated …
The study suggests that RPA impacts on procurement in operational, organisational and relational terms. Furthermore, we identify the motives and challenges when …
This study examines the complex dynamics involved in the digital transformation of the procurement department of a public health care organization in the Middle East. It sheds …
In this article, I would talk about Supply Chain Digitalization with a focus on the procurement side - Procurement 4.0. I’ll run through a brief case study of a large process manufacturer in Asia to explain the points in a relatable manner.
Procurement leaders must be aware of these functionalities; build knowledge about digital procurement technologies, the technology providers, and practical use cases; and gain insights into the benefits and barriers …
The book demonstrates this through a detailed case study of procurement digitalization as a site of unregulated technological experimentation. The book proposes an …